Addressing Artificial Intelligence-Based Hiring Concerns
As scrutiny of AI grows, HR vendors work to add transparency and mitigate potential bias.
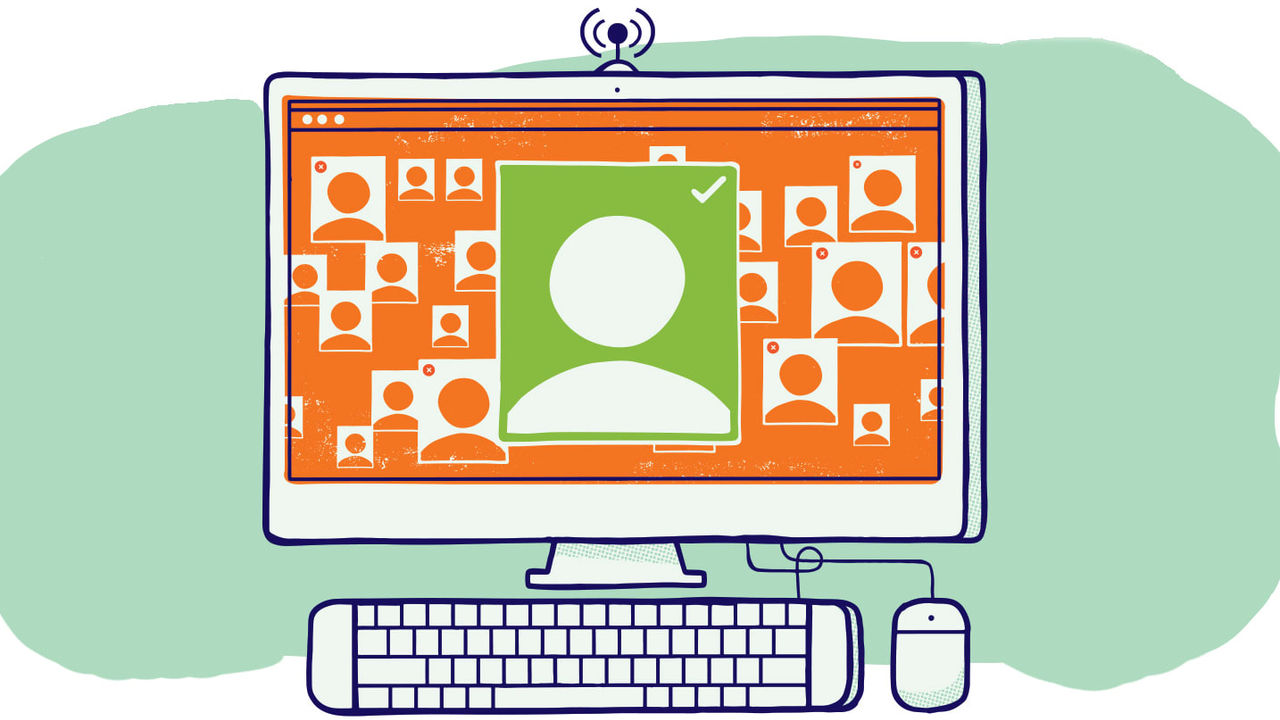
The honeymoon is over for the use of artificial intelligence in human resources. The introduction of a bevy of new artificial intelligence (AI) tools by industry vendors over the past few years was met with a buzz, and it was embraced by HR practitioners seeking to use machine-learning algorithms to bring new efficiencies to recruiting, employee engagement, shared services, learning and development, and other areas of HR.
But as the use of AI has grown, it has attracted more attention from regulators and lawmakers concerned about fairness and ethical issues tied to the technology. Chief among those concerns are a lack of transparency in the way that many AI vendors’ tools work—namely that too many still function as “black boxes” without an easily understood explanation of their inner workings—and that machine-learning algorithms can perpetuate or even exacerbate unconscious bias in hiring decisions.
This increasing scrutiny has manifested itself in a flurry of legislation and regulatory actions designed to create greater oversight of the use of AI in human resources. In January, the state of Illinois signed into law groundbreaking legislation regulating the use of AI in video job interviews. The law requires companies to provide notice to candidates that the technology will be used to analyze their video interviews, explain to candidates how the AI works and obtain candidate consent to be evaluated by AI before any interview takes place.
New Jersey and Washington have since introduced related legislation, and, in February, New York City introduced its own bill designed to regulate the use of AI in hiring, compensation and other HR-related decisions. If the bill is adopted, experts say it would prohibit the sale of AI technology to companies in the city unless the tools have been previously audited for bias.
These actions by lawmakers follow on the heels of steps taken by regulatory bodies to investigate the use of AI tools in the workplace. In late 2019, the Electronic Privacy Information Center (EPIC) filed a petition asking the Federal Trade Commission to investigate the use of AI by vendor HireVue, a leading provider of video-interviewing technology.
EPIC, a public interest research center in Washington, D.C., charged that HireVue was not adhering to international and national standards of fairness, transparency and accountability in the use of its AI-driven interviewing tools. EPIC claims the unregulated use of AI causes harm to job candidates, who are subject to “opaque and unprovable” decision-making in employment and other decisions.
“Some vendors in the past have banked on the mystery and hype of AI as a way to sell their products,” says Matissa Hollister, an assistant professor of organizational behavior at McGill University in Montreal who studies the use of artificial intelligence in the workplace. “But I think they’re seeing increased pressure to be more transparent in what they do.”
Building in Transparency and Fairness
In the wake of this heightened scrutiny, some vendors are taking steps to add transparency and fairness to their AI products. Kevin Parker, CEO of Hire-Vue, says the company’s video-interviewing solutions now include a more detailed what-to-expect screen for job candidates in the spirit of giving them more insight into AI’s role in the evaluation process.
“The screen explains what the online interview will entail, whether AI will help evaluate the candidate’s responses and how the hiring company will use the evaluation as part of their recruiting process,” Parker says.
Experts say many vendors can increase the transparency of their algorithms for HR buyers. Ben Eubanks, principal analyst of Huntsville, Ala.-based Lighthouse Research, an HR research and advisory firm, and author of Artificial Intelligence for HR (Kogan Page, 2018), says vendors can take simple steps to make their AI tools much more understandable.
‘We are not interested in building technology that adds ambiguity as to why a given applicant was selected or rejected by the system.’
Frida Polli
“Most of these AI tools are positioned as infinitely intelligent, but they hinge on a handful of key signals that machine learning is able to use for prediction,” Eubanks says. “Since that’s the case, it would take less than an hour with free tools to create a GIF that shows users how the AI system considers different factors and makes its recommendations.”
Eubanks says vendors often have concerns about sharing their algorithms’ inner workings because they fear it could help candidates game their prehire assessment systems. “But if the AI tool’s recommendations are based on the profile of a high-performing employee or some other objective assessment, there’s no real way to cheat,” he says, “so nothing is gained by keeping the algorithm private or secret.”
Some vendors have long committed to making their use of algorithms transparent and understandable for HR buyers. Pymetrics, a New York City-based provider of pre-employment assessments that use neuroscience and AI to help match candidates to jobs, ensures that its clients grasp the underlying mechanics of its AI assessment models before those models are deployed to evaluate real candidates, says Frida Polli, the company’s co-founder and CEO.
“Given that hiring decisions can have enormous implications for people’s lives, we are not interested in building technology that adds ambiguity as to why a given applicant was selected or rejected by the system, as is the case with ‘black box’ algorithms,” Polli says.
Pymetrics also strives to be fully transparent with the candidates who take its assessments, she says, giving them a detailed follow-up report about their social, emotional and cognitive profiles.
Eyal Grayevsky, co-founder and CEO of San Francisco-based Mya Systems, a provider of a conversational AI platform that automates multiple steps in the recruiting process, says creating a clear understanding among HR buyers of how the company’s machine-learning techniques work has long been a top concern for him.
“Clients have the ability to review, edit and approve content used prior to deploying our solution,” Grayevsky says, referring to Mya’s AI-driven conversations with clients’ job candidates using natural language processing to pre-screen applicants or respond to queries, such as frequently asked questions. “We then offer full transparency into why each candidate was shortlisted and [his or her] resulting status. A transcript is sent back into the client’s applicant tracking system, offering visibility into each candidate conversation.”
How Vendors Can Mitigate Bias in AI Tools
Recruiting experts, academic researchers and AI vendors interviewed for this article offered ideas on how providers of artificial intelligence tools can help mitigate potential bias in their algorithms. These are their key points:
Take technology-based cues. Ben Eubanks, principal analyst of Lighthouse Research, an HR research and advisory firm, says the use of technology-based “nudges” to help promote balanced decision-making on recruiting teams is one of the more promising developments he has seen. For example, when a recruiter is reviewing resumes to create a slate of candidates, an algorithm might recommend a female or minority candidate to add to the slate to help ensure equal consideration, Eubanks says.
“The conversation around unconscious bias is a big one, and the truth is we can’t train it out of ourselves,” he says. “The best bet is to have people or tools like algorithms there to help us be more aware of our decision-making and to provide suggestions and prompts so that we can do the right thing in the moment.”
Scrutinize the data. Frida Polli, CEO of assessment provider Pymetrics, says vendors should be more thoughtful about the type of data they include in AI-based hiring models.
“Metrics that are highly correlated with demographic identity are never going to be the best option for achieving fair outcomes,” Polli says. One reason Pymetrics is able to “de-bias” its AI-driven assessment models is because the traits the models measure—things such as memory and risk taking—are more evenly distributed across the human population, she says.
“Unfortunately much of the data that’s used in conventional hiring processes, like standardized test scores and alma maters, has the potential to exacerbate historical patterns of inequality,” Polli says.
Create diverse development teams. Eyal Grayevsky, CEO of AI platform provider Mya Systems, says hiring a diverse engineering and product development team, training it on unconscious bias, and ensuring that product design decisions are made to consciously reduce bias are important steps to ensure that algorithms remain bias-free. Be thoughtful about who you hire for your team, Grayevsky says, and look for people with different backgrounds, genders, ethnicities and years of experience. –D.Z.
Mitigating Potential Bias in Algorithms
One of the biggest concerns of regulators and legislators is that AI-driven recruiting tools can perpetuate bias in hiring processes. Experts say decisions made by human recruiters about applicants have long been fraught with their own unconscious bias, and, because the datasets used to train AI systems are based on human decisions, the resulting algorithms could be just as likely to encourage discriminatory choices or disparate impact unless this issue is mitigated.
For example, an AI tool trained on a dataset in which human recruiters had avoided hiring graduates from women’s colleges for certain roles will perpetuate that same bias in a machine-learning algorithm.
Some vendors have increased efforts to validate and audit their algorithms to protect against such bias. Parker says HireVue employs a validated bias-mitigation process based on the standard set in the U.S. Equal Employment Opportunity Commission’s Uniform Guidelines for Employee Selection Procedures. Those guidelines are designed to protect against hiring practices that have discriminatory effects on protected groups.
Parker says HireVue is further guided by standards outlined in the Society for Industrial and Organizational Psychology’s principles for the validation and use of personnel selection procedures, and by other professional standards for the design of testing programs. “We’re committed to the continual improvement of our technology and regularly test our models to ensure a level playing field for all people regardless of gender, race or age,” he says.
Regulators and legislators have focused much of their attention on a function of HireVue’s AI that evaluates candidates’ facial expressions and mannerisms in recorded video interviews. The complaint filed by EPIC claimed that such facial-recognition techniques often analyze emotions differently, based on race, for example. EPIC believes that the system could unfairly score candidates based on prejudices related to their race, gender or sexual orientation.
Parker claims that what candidates say in HireVue video interviews—their word choices and the language of their responses—represents the “overwhelming majority” of what is analyzed by the company’s assessments.
“In most interviews and assessments, the candidate’s appearance, how they change their facial expressions, and their mannerisms have no impact on the assessment,” he says, “since they’re not relevant to understanding a candidate’s answers to the interview questions or the competencies measured for a particular job.”
Parker says HireVue now also seeks third-party audits of its algorithms. Experts say such audits can help create confidence among HR buyers that the algorithms they’re purchasing will be free of bias, but most agree that the audits aren’t a panacea.
“Third-party audits might help alleviate fears or minimize perceived risk for buyers,” Eubanks says, “but most buyers still need to be educated on how the algorithm makes decisions in plain language in order to really grasp the significance of such an audit.”
Polli believes third-party audits are a critical tool for promoting public trust in AI and incentivizing vendors to advertise their products genuinely. “We’re currently participating in this very type of process with an academic research team and look forward to sharing the results in the near future,” she says.
Polli believes one of the principal goals of using AI-based hiring assessments should be to reduce the bias that exists when human recruiters screen candidates. “It might be impossible to remove bias from the human brain, but our experience has shown that it is very possible to mitigate bias in algorithms,” she says. “Auditing algorithms is therefore the crucial process we use to make sure improvements in fairness are actually achieved.”
Peter Cappelli, a professor of management at the University of Pennsylvania Wharton School who specializes in HR practices and co-authored a 2019 study with two Wharton colleagues titled Artificial Intelligence in Human Resources Management: Challenges and a Path Forward, says the best way for vendors to validate their algorithms is by using a client’s own data.
“That’s the only way to provide evidence that the algorithms don’t have adverse impacts as well,” Cappelli says. “Showing that algorithms predicted good performance or good hires in company X does not protect their use in company Y. Most of what I still hear is just arguments about why an algorithm should predict an outcome.”
Dave Zielinski is a freelance business writer and editor in Minneapolis.
Illustration by Michael Korfhage.
An organization run by AI is not a futuristic concept. Such technology is already a part of many workplaces and will continue to shape the labor market and HR. Here's how employers and employees can successfully manage generative AI and other AI-powered systems.