Using AI to Improve Engagement Surveys, Continuous Feedback
A Q&A with Ultimate Software's Armen Berjikly
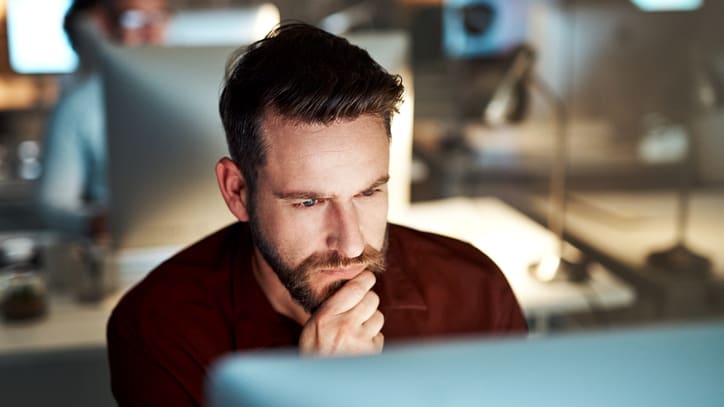
Improving employee engagement is at the top of many human resource leaders' to-do lists. But combing through an ever-growing amount of engagement survey data to extract actionable insights can be overwhelming.
So industry vendors have created artificial intelligence (AI) tools designed to automatically analyze survey data to pinpoint themes and characterize the meaning of words or phrases. Tools like natural language processing (NLP) can save HR time and generate more-useful data along the way.
SHRM Online spoke with Armen Berjikly, senior director of growth strategy for Ultimate Software in Weston, Fla., during the HR Technology Conference & Exposition for his thoughts on the state of NLP technology today, the pros and cons of using AI to analyze engagement survey data, and the importance of developing a code of ethics for using AI in HR.
Prior to working at Ultimate, Berjikly was the founder and CEO of Kanjoya Inc., a workforce intelligence company that pioneered advancements in NLP technology dedicated to understanding human emotion. (Kanjoya was acquired by Ultimate Software in 2016.)
SHRM Online: Artificial intelligence has made inroads into many areas of human resources. Where do you see new areas of opportunity for AI within HR going forward?
Berjikly: I think we are at the beginning of a very long journey that is virtually unlimited. Applying AI to continuous feedback—apart from regular engagement surveys—is one area for near-term opportunity. We believe it should be easier to give quality feedback to people to help them better understand what they're doing well now and how they can improve in the future.
For example, NLP might be used to analyze unstructured data—such as text—that comes as a piece of feedback from a manager [or] a peer or in a performance review to make it more understandable and useful. The use of "guardrails" in that process could help people write more-valuable feedback, avoid toxic language or use more-actionable terms when describing or evaluating others. For example, does describing someone as "fun" help them improve in any meaningful way? NLP tools could help managers determine if their feedback is in the right realm of quality to help others truly grow and improve.
The potential to dig deeper into human language and close the gap between what is said and what is actually meant is profound. This goes beyond simply identifying positive and negative language to deciphering different shades of emotions. If someone is excited about a new product or someone is worried about pay equity, for example, sentiment analysis will pick it up in a very detailed way. A greater level of specificity leads to more-accurate conclusions.
If you work in HR, you are often under-resourced and understaffed. HR is usually good at making tough people decisions under such duress, but it needs more time- and cost-efficient ways to apply meaningful data at scale to improve the decisions it makes about who to hire, how to better engage people, who to pay more, who to promote and so on. The goal should be to use AI to help HR make decisions with more confidence and more insight and with less bias.
SHRM Online: What can you tell me about developing a code of ethics to follow when using AI, especially with growing concerns about the technology perpetuating unconscious bias in people decisions or even putting HR professionals out of work?
Berjikly: Developing a code of ethics around AI has been an important touchstone for me. While HR professionals may not have as much at stake in their decisions as, say, emergency room doctors, they are enablers of crucial people decisions.
We can't take the same cavalier approach other industries or functions have where it concerns advanced technology. The traditional approach is almost to deify the technology and say, "We need to let it go where it goes and fix any problems that occur after the fact." We can't do that in HR. We can't be reckless in letting technology be the sole driver of processes.
It's important that AI providers be transparent with customers about what they're doing with their algorithms and why they are doing it. Our goal is to use technology exclusively to help people make better decisions, not to replace them or their roles. The idea isn't to believe you can now run HR with a joystick and skilled people are no longer needed.
We build "gate checks" into our machine learning algorithms to regularly test how they're performing. When an algorithm goes out "into the wild," it ingests and learns new data and theoretically should be getting smarter. The concern is that the algorithm can sometimes get smarter in ways you don't anticipate. It can pick up any bias in either its developers or its users.
Algorithms can actually score higher and higher on accuracy while at the same time undermining a longer-term ethical goal because the machine is trying to be really good at copying decisions historically made in the organization that were considered sound but that may have been questionable. So it's important to put in gate checks as algorithms evolve from version one to version two and so on to evaluate their performance. You can't start thinking that technology is infallible because it will lead to unanticipated consequences.
SHRM Online: What can be done to help organizations achieve more return from the investments they make in measuring and improving employee engagement?
Berjikly: We still too often ask employees to speak our language when it comes to HR technology and surveys rather than us speaking their language. If we can figure out what is really going on in employees' work lives by deciphering the true meaning of their language, it can help managers take steps to make meaningful changes.
In some cases, there's no need to invent new AI applications to make this happen. The technology already exists but just needs to be harnessed and orchestrated in new ways. One area for opportunity is improving integration between HR datasets. Consider the lack of connection between performance reviews and employee engagement surveys in most organizations. When you merge those two datasets, you get a more complete and accurate view of employees.
In a performance review, for example, someone might be perceived and evaluated as a bit aloof, unfriendly or difficult to work with in a team environment. But if you consider only that information on its own, you might get a skewed perspective. Instead, if you factored in survey feedback from the individual being reviewed, it might indicate that they're an introvert; that ever since the company switched to an open-office design, they feel they get interrupted more often and can't get their work done; or that they're uncomfortable being in the limelight.
Integrating those two datasets might allow a manager to realize that this person used to be a very good and productive employee, but the company dynamic has changed. Perhaps they can still work at a high level, but it might mean making some adjustments like allowing them to work from home a few days a week.
Dave Zielinski is a freelance business writer in Minneapolis.
Advertisement
An organization run by AI is not a futuristic concept. Such technology is already a part of many workplaces and will continue to shape the labor market and HR. Here's how employers and employees can successfully manage generative AI and other AI-powered systems.
Advertisement